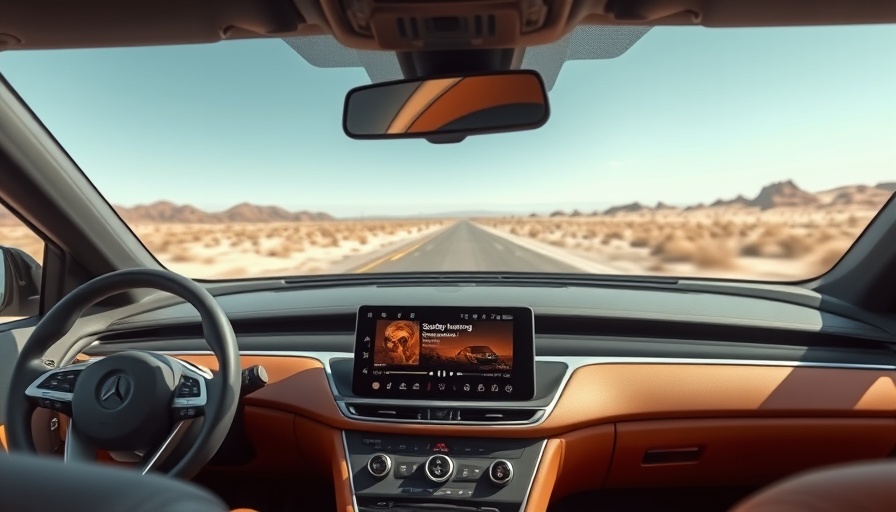
Challenging the AGI Benchmark: A Closer Look at ARC-AGI
Artificial General Intelligence (AGI) has been a long-standing enigma in the AI community, and a recently pivotal benchmark, known as ARC-AGI, introduced by Francois Chollet in 2019, aimed to quantify how close we are to achieving it. This test, the Abstract and Reasoning Corpus for AGI, asks whether AI can develop new skills beyond its initial data training. Yet, instead of symbolizing a breakthrough, our latest performances on this test reveal potential fundamental flaws in its approach.
Progress or Pitfalls? Examining Recent Advances
Initially, AI models could solve only a third of ARC-AGI tasks. However, with a significant improvement to 55.5% accuracy, this recent leap was due to a $1 million challenge co-initiated by Chollet and Mike Knoop. Despite this leap, it falls short of the 85% threshold needed to claim human-level intelligence. Critics argue this progress might be misleading since many solutions rely on brute-force techniques rather than genuine problem-solving, casting doubt on ARC-AGI's effectiveness as a true measure of AGI.
Diverse Perspectives and the Path Forward
The debate around AGI importance is expanding, as some experts suggest the test has been oversold. One OpenAI staff member controversially claimed AGI could already be a reality, depending on how it's defined, highlighting the contentious nature of AGI itself. Meanwhile, stepping away from large language models (LLMs) and their limitations in generalization remains crucial. Future efforts may need to explore alternative AI models that go beyond pattern recognition.
What Does This Mean for the Future of AI Development?
Understanding the nuances of ARC-AGI's results is vital. It hints that while we can refine AI systems to perform specific tasks better, genuine AGI—teaching machines to learn and adapt like humans—requires innovation beyond our current benchmarks. This realization emphasizes the need for fresh approaches and foundational changes in AI research.
Write A Comment