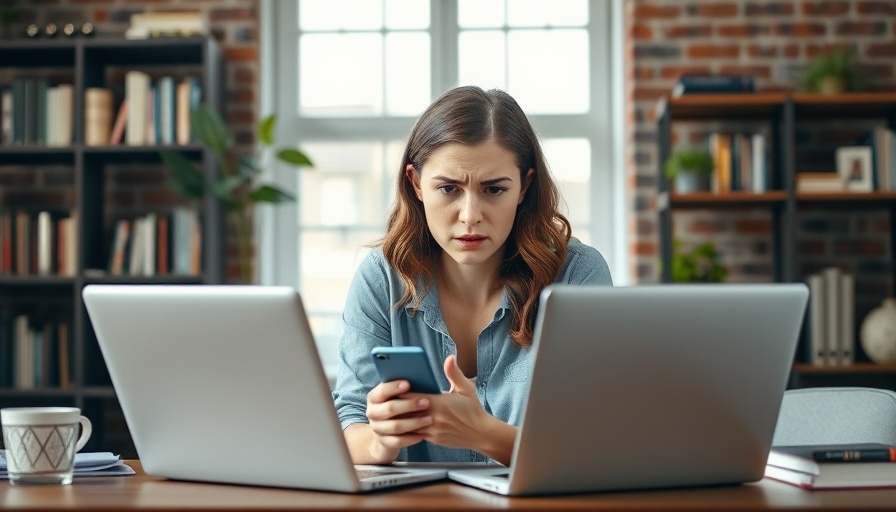
Understanding the Issue: Google's New Guidelines for Gemini Ratings
In the fast-paced world of Generative AI, systems like Google's Gemini are constantly under scrutiny to ensure accuracy and reliability. Recently, however, Google has introduced a new guideline that requires contractors to rate AI-generated responses even when these fall outside their area of expertise. This decision has sparked concern among those tasked with evaluating highly technical content.
Potential Impacts on AI Accuracy
The new mandate affects contractors working with GlobalLogic, a firm outsourced by Google for tasks related to Gemini. Previously, these contractors had the liberty to bypass questions outside their knowledge realm, such as those requiring specific medical or scientific understanding. The change now presses these evaluators to rate as much as they comprehend, and simply note their lack of expertise where relevant. Critics argue that this diminishes the quality and reliability of Gemini, especially on intricate subjects like healthcare or technology.
Diverse Perspectives: Concerns and Implications
While the directive aims to streamline the evaluation process, it also raises valid concerns about the integrity of the AI's responses. Contractors have voiced worries, questioning the benefit of a policy that seems to prioritize quantity over quality evaluations. "I thought the point of skipping was to increase accuracy by giving it to someone better?" expressed one contractor, highlighting fears that the AI's accuracy could be compromised.
Historical Context and Background
Earlier guidelines explicitly allowed skipping prompts that required special domain knowledge, aligning with a system designed to hone AI precision through expert feedback. This shift away from targeted expertise paves new ground in AI development practices, reflecting possible pressures to expedite AI training despite potential sacrifices in quality control.
Future Predictions and Trends
Looking ahead, this change may prompt reconsiderations in how AI firms leverage human resources to refine machine learning models. As the field advances, reliance on specialized knowledge will likely remain critical to AI's success. Companies may need to balance the urgency of AI development with maintaining trust and accuracy in their AI products, possibly leading to industry-wide discussions and revisions in AI training methodologies.
Write A Comment