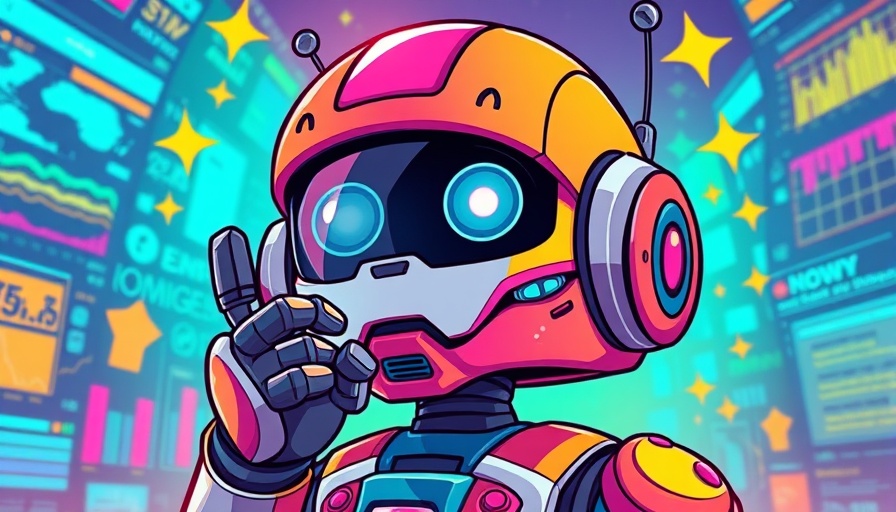
Creating Custom Language Models Without Breaking the Bank
In the rapidly evolving world of language models, businesses of all sizes need a language model (LLM) that understands their unique data sets. Whether you're a small startup or a large corporation, optimizing an LLM to fit your business data can transform how you engage with technology and streamline operations. Here, we explore four strategies to craft a specialized LLM while managing resources effectively.
1. Start with Simple Prompting Tactics
The easiest gateway into creating an LLM is through prompting. Just like composing a question, prompting involves crafting the right queries to guide the LLM. Start with straightforward prompts and evolve by including few-shot examples or chain of thought prompting. This approach is time-efficient and can often achieve the desired outcomes without complex interventions.
2. Enhancing Outputs with Retrieval Augmented Generation (RAG)
RAG blends prompting with data retrieval to supply the language model with additional context. By indexing chunks of domain-specific data, businesses can enrich model outputs with precision. This approach helps reduce errors and ensures the LLM provides information backed by comprehensive data, promising clarity and accuracy.
Future Predictions and Trends
As LLM technology develops, businesses can expect finer-tuned models with increased efficiency and application scope. Leveraging techniques like LoRA or full finetuning will likely become industry standards as they offer enhanced performance at reduced costs. Predicting the emergence of more sophisticated, user-friendly platforms, we advise executives to stay informed and adaptable to incorporate these trends for a competitive edge.
3. Exploring Cost-Effective Finetuning with LoRA
LoRA presents a savvy approach to finetuning. By breaking down complex weight updates, it reduces the number of parameters needing adjustment. This means businesses can fine-tune models to their specific needs without imposing significant computational costs, maintaining model performance while minimizing overhead.
4. Full Finetuning for Maximum Customization
If other methods don’t meet expectations, full finetuning is the comprehensive method for tailoring an LLM to your specific domain of expertise. Though more resource-intensive, this involves training the model with a curated data corpus to further refine language understanding capabilities. This approach is invaluable for businesses holding vast repositories of unique data.
Write A Comment